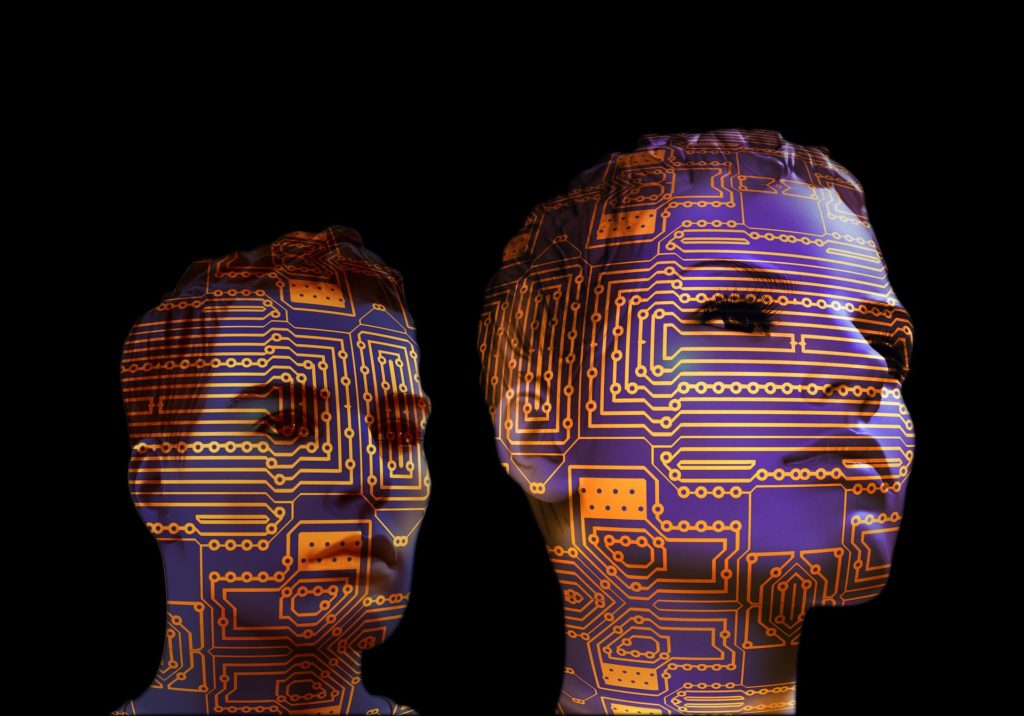
As AI technology continues to gather momentum, 150sec spoke to Naila Murray, Scientific Director at Naver Labs Europe to gain insight into the challenges standing in the way of the technology’s progression.
Naver Labs Europe is the biggest AI industrial research centre in France. The precursor to Naver Labs was part of Xerox for more than 20 years. Now, under the ownership of South Korean internet company Naver since 2017, the centre has broadened its sphere of interest from a core competence of text and document understanding. AI has proven to be good at recognizing images. However, comprehending text and documents through AI has been far more challenging.
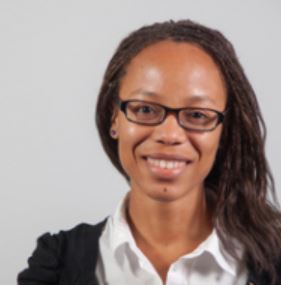
Recently, the company has expanded into areas more adjacent to robotics, 3D vision and geometry, Murray told 150sec. The AI research that is being pursued is very broad. This is something that she believes is one of the centre’s strengths, because “AI benefits a lot from interdisciplinarity.”
1. The data issue
Considering the current challenges facing AI, the Lab’s Scientific Director explained that the enormous volumes of data needed for AI modelling has been a known issue for quite some time. People in the industry who have asked themselves where this data use is going feel that it’s not sustainable. The question that is being asked by many within the industry is how can AI computation and modelling be achieved with less data? According to Murray, coming up with AI systems that are not data hungry “is a very real and challenging problem.”
The nature of the problem is compounded further in domains where it is not possible to collect the amount of data currently needed for AI computation and modelling. Scientist Murray uses the example of medical applications, a field in which it is almost impossible to cover entirely. “The point is that it is never going to be possible to cover all the minute diseases that you might want to tackle”, Murray added.
2. Disadvantaged areas
In Europe, the issue of acquiring the necessary volumes of AI data for computation and modelling is very important. In the US and China, the common use of one main language within each region means that both have a lot of data.
“There are many countries, many cultures and many languages that don’t have [AI data access].”
Naila Murray, Scientific Director, Naver Labs Europe.
This is not the case in regions with a mishmash of language use such as Europe. Therefore, locations outside of the major AI research centres are considerably disadvantaged. As Murray puts it, “there are many countries, many cultures and many languages that don’t have [AI data access]”. The knock-on effect is that there are many cases in which fundamental AI techniques cannot be applied because they are dependent on volumes of data and annotated data.
3. Scaling AI
Another issue which has come to the fore is the computing power requirement and associated energy cost implicated with machine learning. OpenAI recently noted that the computing power required for AI-based techniques is doubling every three and a half months. Research published earlier this year also found that the training of a neural network creates a carbon dioxide footprint of 284 tonnes. That’s the equivalent of five times the lifespan emissions of a typical car.
“You can have more computation, but do you want to also be running the servers and take the energy hit that you need in order to complete some of these models?”
Naila Murray, Scientific Director, Naver Labs Europe
Facebook’s Head of AI – Jerome Pesenti – recently stated that this issue may mean that AI has hit a wall when it comes to scaling. Murray too has concerns surrounding sustainability and the current application of the technology. “You can have more computation, but do you want to also be running the servers and take the energy hit that you need in order to complete some of these models?” she asked. This level of computation comes with a trade off as the levels of energy usage that come with it are high.
4. Setting the right objectives
Setting the right objectives for the machines to learn may seem very simple. However, Murray points out that it is anything but. She cites the example of a robot that’s tasked with ‘finding the kitchen’ within a house. It seems very simple but there are many unstated constraints that we want robots to hold that are not expressed within existing AI systems.
It’s very hard to formulate mathematically but that’s what Murray believes current machine learning paradigms need.
5. Regulatory & strategic challenges
There are also challenges in terms of how governments approach the technology, regulate the space and educate citizens regarding its use. Murray complements ‘AI for Humanity‘ – a strategic approach taken by the French government towards the technology. This approach proposes the establishment of an ethical framework to advance the use of machine learning within France. She feels that further work is required when it comes to the establishment of data privacy frameworks relative to AI. This would provide users with confidence and as a consequence, advance the ability of the industry and its technological ability to progress.
These are all major factors affecting the development of AI in Europe. However, given the depth and breath of the industry and the enormous implications of the technology, it’s likely that ways can be found to overcome these difficulties in the medium term.