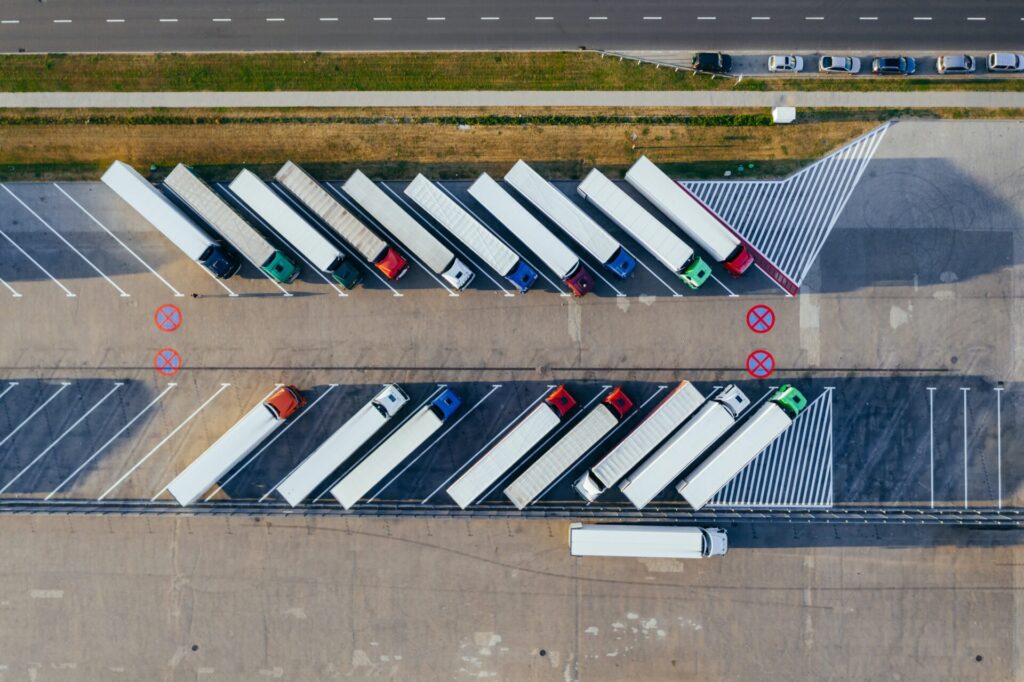
Guest author: Hristo Hadjitchonev, Product Manager at Transmetrics.
Practically 95% of any human reaction is a form of prediction. From how one gets dressed to the chosen commute, every decision is guesswork based on parameters such as the weather, traffic, and time of day.
Yet, as inventory forecasters predict demand on multiple levels, the number of external factors impacting their decisions also skyrocket, making it extremely difficult for humans to make the predictions.
Robust inventory management is the unsung hero in logistics—and it’s encouraging the global supply chain AI market to reach $17.5 billion by 2028. But it’s dependent on one thing: Accurate forecasting.
Logistics providers and data teams today need to be hot on understanding the power of data, what sources they require to meet their objectives, and how to maintain its quality.
With that in mind, let’s explore how paying more attention to data quality and advanced analytics can optimize inventory forecasting and improve operational efficiency.
Why Accurate Data is Essential in Forecasting
McKinsey reports that applying AI-driven forecasting to supply chains reduces errors between 20% and 50%. But the limited usefulness of data collected remains the top barrier to successful implementation, keeping the rate of AI adoption since 2018 at a low 6%. With the right data and tools, inventory forecasters can predict anything from how much time they have to release trending products to the risks and costs of missing out on the opportunity.
Take fast-fashion brands, for example. They distribute up to 20,000 clothing stock-keeping units (SKUs) across ten countries in the EU, where price sensitivity and sizes vary per location. Then the new Barbie movie is released, and suddenly everyone wants to wear pink. That’s over 200,000 SKUs to consider barbie-fying.
While stores like Gap are ahead of the game in creating a Gap x Barbie collection, others are apprehensive, knowing that the merchandise will end up on sale if movie ratings are low.
Inventory forecasters need to weigh everything from budget to the predictability of movie success—and they want these figures yesterday. With accurate data regarding operations, such as delivery speed, workers available, and vehicle capacity, alongside external inputs, including consumer trends, AI-powered analytics tools can help inventory teams make data-driven decisions instantly.
AI for Data Collection and Storage
Many organizations possess sufficient data to leverage the benefits of AI-driven forecasting: A quality forecast could be achieved on 80% internal and 20% external data. The trick is ensuring that data is collected, stored, and visible to inventory teams.
And this truth is something data champions like Gather AI and IFM are more than aware of. For stores to better support customer fulfillment and omnichannel retailing, the two companies have started putting drones to work as a method of tracking warehouse inventory.
From counting parcels to spotting retail theft at checkout, computer vision AI technology tools can detect and track objects and SKUs as they move through the warehouse or store. This helps provide the continuous and reliable updates needed for making real-time data-driven decisions while removing room for human error.
Automating data collection, such as the number of items on shelves and the locations of particular pallets, helps inventory teams understand their operations and needs in real time. While storing operational, financial, and external data required to meet inventory objectives in central repositories enables data teams to capture a holistic view.
That’s why retailers and logistics companies must devote more time to their data collection and storage methods, looking at how they can increase data visibility. This includes ensuring strict access controls and encryptions to protect data and keep it in the hands of those with authorization.
AI for Data-Driven Inventory Forecasting
At the analytical stage of the data lifecycle, AI forecasting tools, fed with continuous and reliable data, can predict sales down to the SKU level. This way, sellers can adjust production levels, manage procurement, and allocate resources effectively. This alignment of inventory with demand reduces handling and storage costs and improves overall operational and financial efficiency.
Here’s how to implement forecasting models with success:
- Define the critical parameters: What business changes could affect the inventory variables? What product expiration periods, storage space, and storage conditions are required? How could staff turnover and ship maintenance affect this? Defining reasonable ranges for these parameters is crucial to avoid creating unrealistic outputs leading to adverse business decisions.
- Apply data smoothing: Unexpected events will likely skew AI predictions. The smoothing technique is a statistical approach to eliminating outliers from datasets. It helps to minimize the variation in data between periods where an anomaly occurred, creating a more representative data set for AI-powered forecasting models to learn from.
Take Covid-19, for instance. The data captured during this time falsely illustrate business as usual and would require smoothing.
- Train what-if simulations with augmented data: If data is incomplete, logistics teams struggle to have an accurate historical representation, and assumptions go awry. For this reason, augmented data—that is ‘synthesized’ or ‘artificial’ information generated from existing data—is used to train AI-powered forecasting models with potential scenarios. The machine can define more data classifications and increase predictability by reading a broader data set.
What-if scenarios are critical in cases of limited data samples, which diminishes confidence in forecasting with accuracy. They are also valuable for addressing distant future uncertainty where it is challenging to predict unexpected events.
Depending on the size of your data, you may choose a simpler AI model or a more complex one where larger data sets and many iterations are available to train numerous parameters.
Harnessing the power of data-driven inventory forecasting is the key to unlocking logistics companies’ full potential in today’s complex supply chain landscape. With robust forecasting models, inventory managers can ensure the right products are available when customers need them, leading to enhanced customer satisfaction, loyalty, and a competitive edge. Organizations can go on to assess market opportunities, plan new product launches, manage product lifecycles, and make optimal supply chain decisions aligned with business objectives.